The Search for Critical Minerals is Going High Tech
In the fall of 2023, Matt Theurer and his team at HyperSpectral, an artificial intelligence company, landed a meeting with the Defense Advanced Research Projects Agency. They had sophisticated technology to pitch to the famed Department of Defense research organization: artificial intelligence software that analyzes the light emitted and absorbed by a given material, then uses that data to pluck the faint fingerprints of, in Theurer’s words, “small, unwanted things” — like E. coli or staph bacteria.
DARPA, though, was also interested in something else. The tool designed to pick out a bacterium on a wall could, they reasoned, also learn about physical material’s properties. Different fields of study can represent the same kind of puzzle, reflected Theurer: “How do you detect that super faint signal when there’s a whole bunch of other mess around it?”
HyperSpectral announced in July that they had made a research agreement to prospect the possibilities. “To understand the broad applicability of artificial intelligence to spectral data,” as Theurer put it. In the future, that could mean using on-the-ground data to ensure that, say, the element germanium is produced domestically and is actually pure. HyperSpectral’s technology could also analyze satellite or drone data to find out where someone might mine germanium on the mainland in the first place.
DARPA’s interest in those questions comes at a time when U.S. officials are concerned about the country’s supply of so-called “critical minerals,” which include nickel, lithium, and dozens of other naturally occurring substances that have been deemed necessary to the economy and national security.
Those minerals, though, are often mined in countries with which the U.S. has, at best, a tenuous relationship, like Russia and China. To protect against the possibility that those nations might cut the supply of minerals that go in phones, fighter jets, solar panels, batteries, electric cars, and computers, the U.S. is scrambling to secure its own raw sources. Without reliable supplies, manufacturing the technologies critical for everyday life — that people use to do work, get to band practice, call their moms, or watch for incoming missiles — could get backed up, resulting in demand outweighing the supply.
But to get those supply chains flowing, the U.S. government, private companies, and researchers must first figure out where to dig for the necessary materials. That might involve analyzing new data from aerial surveys, as HyperSpectral’s AI technology could, or using artificial intelligence to survey existing, older data, pulling obscure signals from the past. And the analysis is legally necessary: The Energy Act of 2020 and the Bipartisan Infrastructure Law have tasked the nation’s geologists with evaluating mineral deposits within the country.
“How do you detect that super faint signal when there’s a whole bunch of other mess around it?”
Learning the locations of those terrestrial ingredients has historically been a low-tech process — albeit one that involves lots of training, expertise, and data — and one handled by civilians. But given the criticality of the problem, DARPA — an agency that invests in high-risk, high-reward projects — is seeking to advance the scientific studies that underlie mining. That involves parsing old, fuzzy, sometimes confusing data, as well as understanding reams of new information streaming in from satellites and aerial surveys. And while AI can help with that analysis, humans — with their geological expertise — still have to be involved.
HyperSpectral’s technology could eventually help them tunnel in that direction, finding or validating mineral supplies using spectroscopy, or the data from their light signatures. But other federal and private research efforts are taking place, including at DARPA, which has recently led a successive suite of programs aiming to use AI to help humans find out what lies beneath Earth’s exterior, and collaborating with other federal agencies, like the U.S. Geological Survey, to do so.
Surprisingly, that subterranean space is still a relative mystery, said Graham Lederer, a geologist at USGS: “We’ve barely scratched the surface.”
The Department of Defense has reason to be concerned about its critical mineral access. In 2023 and 2024, China imposed export controls on ingredients necessary for semiconductors, computer chips, and defense technology like night-vision goggles, infrared sensors like those that guide missiles, and nuclear weapons.
The supply choke-off can come as a response to geopolitical events, as when China stopped sending rare-earth metals to Japan during a conflict. Similarly, Russian President Vladimir Putin, in response to sanctions on his country, has said he might curb exports of uranium, titanium, and nickel — the latter two of which go in everything from batteries to detergent to medical devices.
Without diversified, native, and validated supplies, the U.S. can’t guarantee access to the material it needs to keep its computers running, and its troops outfitted. Nor will it necessarily have the material to go green, since critical minerals are used in products like solar panels and electric cars. “The energy transition is really a minerals transition,” said Douglas Wicks, a program director at the Advanced Research Projects Agency–Energy, or ARPA-E. It’s the Department of Energy’s version of DARPA, and another partner in DARPA’s prospecting programs.
“We’re moving from the era of Big Oil to Big Metals,” Wicks added.
The U.S. wants to turn its focus inward by finding and digging out those metals at home, rather than outsourcing the task as it has in recent decades. (This outsourcing has been due in part to environmental regulations that made domestic mining less tenable, as well as public sentiment regarding mining-related pollution.) But that situation will only change if geologists know where in Earth’s crust those metals are lodged, a task that’s harder today than it was in, say, the 1800s, since many obvious deposits have already been scraped. Meanwhile, demand is growing: “The amount that we’ve mined so far pales in comparison to what we’re going to need in the future,” said Wen Song, head of the Critical Minerals Working Group, a collaboration that brings together mineral research, technology, and workforce development at the University of Texas at Austin.
Historically, gathering that information has been a manual process, with prospectors surveying the cliffs and outcrops that poke from Earth’s surface, inferring what may be underneath, and doing initial digging to get a sense of the underground truth. That has resulted in reams of data. The USGS, for instance, has more than 100,000 geologic maps, which lay out the planet’s rock stratifications and structures. Just 10 percent of those have been georeferenced — meaning their written coordinates have been matched up with the actual coordinates of Earth. And of those, only half are digitized and ready for analysis.
“We need to be able to identify and locate critical mineral resources much faster than they have in the past,” said Wicks. Congressional requirements mean the USGS has to assess all the critical mineral resources, and — barring any drastic change from a potential Republican-controlled Congress — the Bipartisan Infrastructure Law gives a deadline of 2032. That deadline has had them sweating. “A typical mineral resource assessment might take a team of geologists — I’ll say, a half dozen people — two years start-to-finish,” said Lederer. And there are 50 critical minerals.
“We’re moving from the era of Big Oil to Big Metals.”
Officials at DARPA understood the importance of enhancing U.S. supply chains and reached out to the USGS, as the mineral specialists. Together, the two agencies realized the job of understanding what useful supplies might be where was a gargantuan task. And they set about whipping some of geology’s old-school methods into the 21st century, using AI to help identify and quantify the desired goods.
And so it was that DARPA and the USGS — in their first public collaboration in recent memory — launched the AI for Critical Mineral Assessment Competition in August 2022, alongside other collaborating institutions. The competition asked participants to use AI to take old geologic maps, whose coordinates may have been in different formats from modern ones, and line them up with the real world, georeferencing them. Then, they had to interpret the actual contents in those maps. That meant taking features from the maps’ legends — like places where people had dug prospecting pits or mine shafts, text, or the boundaries of a particular rock formation — and extracting them automatically. While HyperSpectral is deploying AI to make mineral sense of digital spectral data, this contest tasked competitors with using the technology to bring similar sense to old, analog data.
But even with today’s technological capabilities — computers can readily identify a face in a crowd — analyzing maps isn’t simple, since symbols and outlines may have blurred with time, their color faded, their meaning distorted. On top of that, according to an email from Justin Gawrilow of Jataware, a software company that competed, every map displays its data slightly differently.
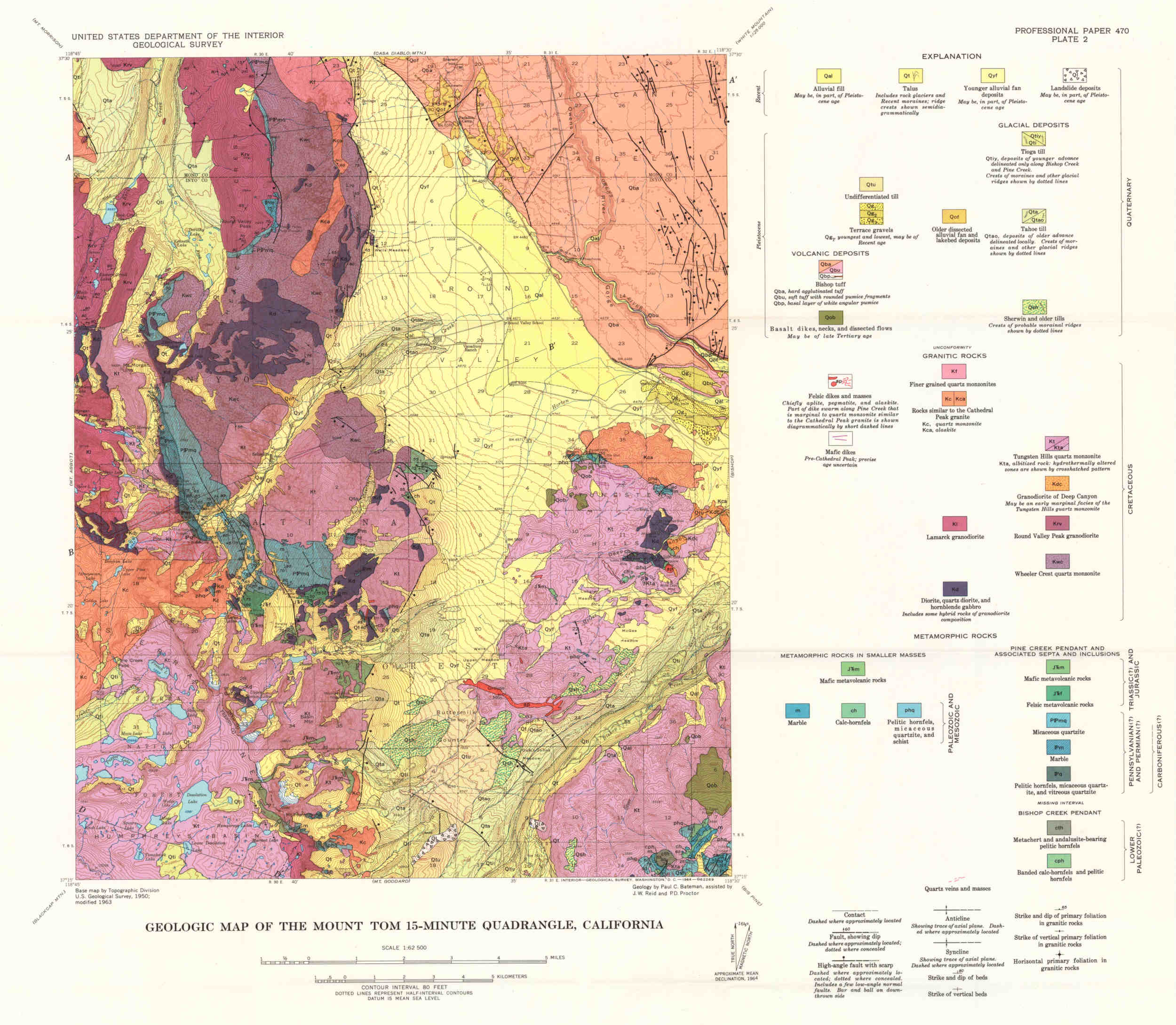
One team, at the University of Southern California, had previously worked on pulling text and the roads from more general maps. One of the team’s Ph.D. students expanded that work to focus on fault lines — geographic features that could reveal the mineralogy beneath. It’s not dissimilar from what HyperSpectral is doing with light signatures: extracting obscured mineral meaning from noisy, unclear data.
Craig Knoblock, executive director of USC’s Viterbi Information Sciences Institute, who served as one of two advisers, says his team used machine learning to show the software what to look for, creating synthetic maps that give the software the right answer, so it can learn what right looks like — to create, for instance, a map with fault lines correctly marked as such.
They also used machine learning techniques to pull together information from databases, journal articles, and mining reports. Those go together into what’s called a knowledge graph — “a graphical representation of some knowledge that shows all the connections between things and uses a standard set of terms to describe all the data,” Knoblock wrote in an email. Information about a single geographical site is linked in the graph.
These teams, and DARPA, live in an ecosystem of others working on similar problems. Song, at the University of Texas, got interested in mineral availability after looking at challenges in the sustainable energy sector in 2019, when she decided to switch her focus away from oil-related research. “That’s when I kind of stumbled on this idea that there’s a lot of these metal elements that are very critical for the function of our devices, and we don’t think about this very much.” Back then, she was the only one on campus directly focused on those metals; now, they have eight affiliated researchers and are planning for a workforce training program.
“The goal of the program was to speed up this process by something on the order of like 1,000 times.”
At the National Energy Technology Laboratory, for instance, scientists recently used AI to unearth a deposit of magnetic rare Earth elements, estimated at 1.2 million tons. The software used geologic data to look for likely deposits near coal supplies, a mineral association that had previously been overlooked. The group produced a “prospectivity map,” suggesting where the desired elements could be found, and then sent mining company Ramaco Resources to confirm that the ground truth matched the model. The results were published last year.
On the commercial side, companies like VerAI and KoBold Metals are mining existing data for new insights using AI. KoBold recently discovered one of the world’s highest-quality copper caches this way. The company, which also scans for new data, is a charter member of Stanford’s Mineral-X initiative. The initiative aims to make mining for the clean-energy transition better than the mining of the past in terms of environmental responsibility, increased efficiency from tools like AI, and engagement with communities that will be affected by extraction. These efforts are all united by the idea that though today’s mineral deposits are harder to spot, there’s plenty of data from which to unearth them — an uncovering that will be necessary if humans are to move away from fossil fuels and keep new technology coming.
DARPA kept its own rock rolling after the initial competition with another program called Critical Mineral Assessments with AI Support, or CriticalMAAS, launched last year. It expanded on the previous work, automating and accelerating the mineral assessments the government is demanding of the USGS. “The goal of the program was to speed up this process by something on the order of like 1,000 times,” said Erica Briscoe, CriticalMAAS’s manager.
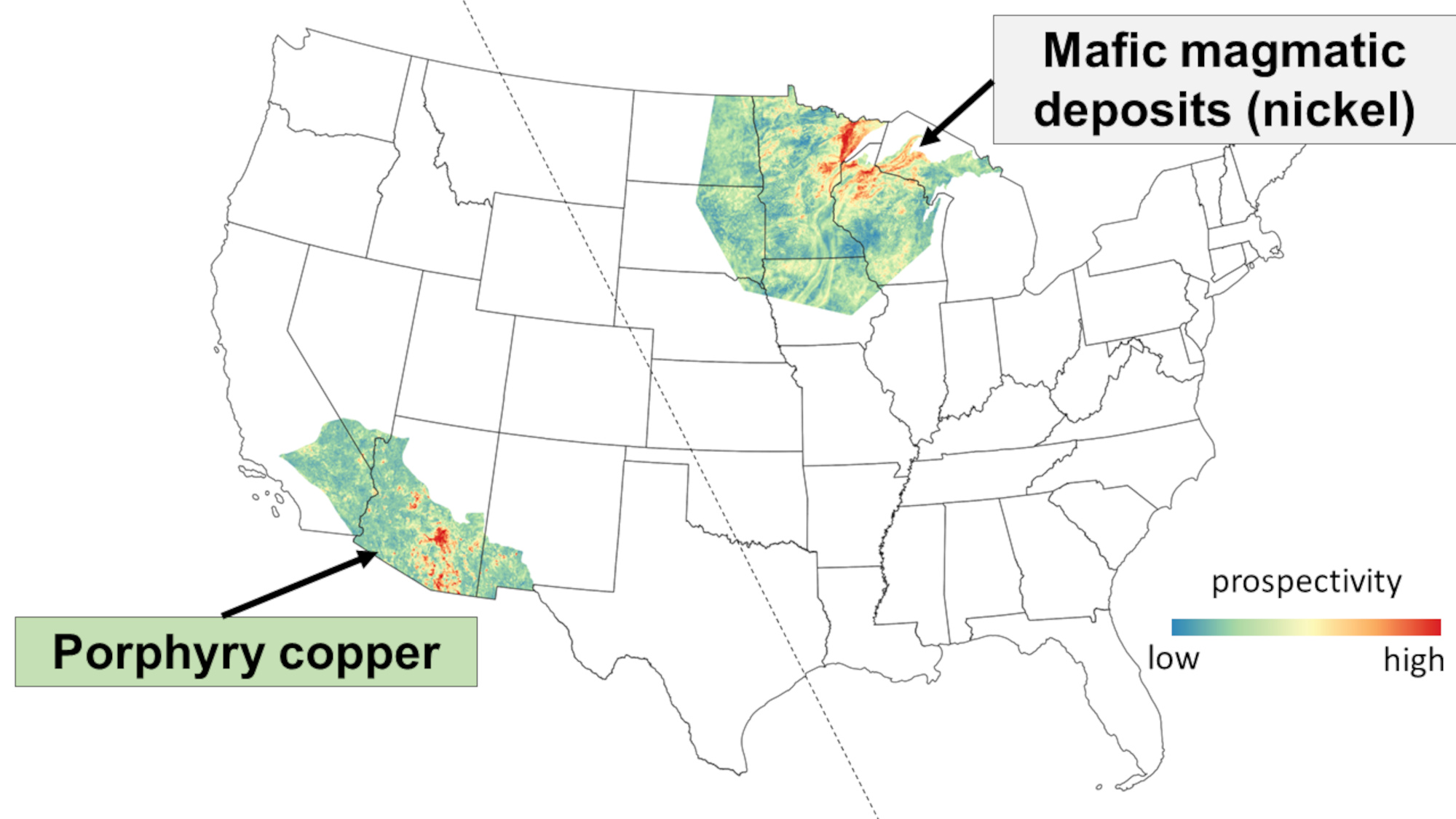
This program is still in process. DARPA hopes developers will be able to take the geologic information they digitize and extract, and use it to make new AI-generated maps indicating the potential location and quantities of particular minerals — and so where the miners of the future might want to slam their proverbial pickaxes, in the name of national security.
One key part of that is understanding where people have mined, say, lithium in the past. Pulled out, that information could be used to train a machine learning system to understand where that mineral tends to be. “That will then allow the learning system to make predictions about where to look for those same minerals,” said Knoblock in an email.
But before anyone actually goes looking, humans will be part of the computational picture. That is, in fact, Jataware’s main role in CriticalMAAS: building interfaces to help humans to either validate or correct the computer work. “AI is great. It can get you a lot of the way there,” said Gawrilow. “It’s not perfect. And so our system that we’re starting to build is aiming to help the human.”
Humans working in the field, alongside their silicon help, are making progress. In a recent CriticalMAAS hackathon, where competitors collaborated in person, Lederer and his colleagues gave the teams old geologic maps and also data from a USGS initiative called Earth MRI. Through Earth MRI, geologists have been gathering new aerial and on-the-ground data of American areas where critical minerals might reside. “Go out and find everything you can about tungsten,” said Lederer, of the mandate.
They did: In about a week, as opposed to two years, the teams’ combined software solution had done a mineral assessment, which was then assessed by humans, on the metal. “We’ve been whittling away this problem with hand tools, and now we’ve just got some really beefy power tools,” said Lederer.
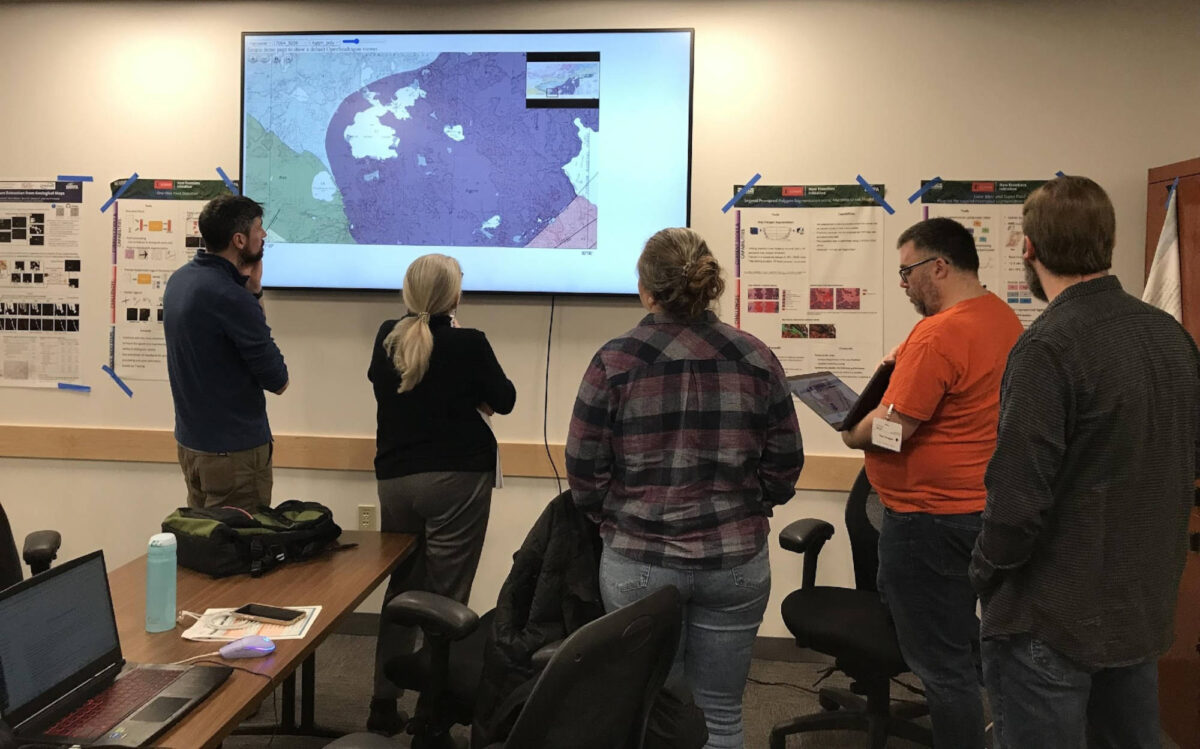
CriticalMAAS researchers gather at the USGS National Training Center in Denver, Colorado, for a hackathon, which addressed a critical mineral assessment for nickel.
Visual: MITRE
In the future, that sort of automated assessment, combining old and new information, might also involve work like HyperSpectral’s. Earth MRI, for instance, is gathering spectral data that could use fresh, computer-generated eyes to spot deposits other prospectors have missed. And the USGS also recently characterized the spectral signatures of critical minerals that were still missing from their catalog.
“Spectroscopy has been around forever,” said Theurer. But the hardware to take detailed data has gotten cheaper at the same time as computing power, while AI has grown more artificially intelligent. What that may mean for minerals remains to be fully investigated.
Theurer said he wants to demonstrate to DARPA what the confluence of hardware and software advancement could mean — what small signals HyperSpectral could see. “It’s just showing them that this is possible.”
Your ability to distill complex concepts into digestible nuggets of wisdom is truly remarkable. I always come away from your blog feeling enlightened and inspired. Keep up the phenomenal work!