Five Questions for Erik Brynjolfsson
In 2014, Erik Brynjolfsson and his MIT colleague Andrew McAfee published a best-selling book, “The Second Machine Age,” arguing that the world had entered a new era of technological advancement. In contrast to the first machine age — the Industrial Revolution, in which machines were limited to physical tasks — this new age would see the rise of artificial intelligence, in which machines would learn to think for themselves.
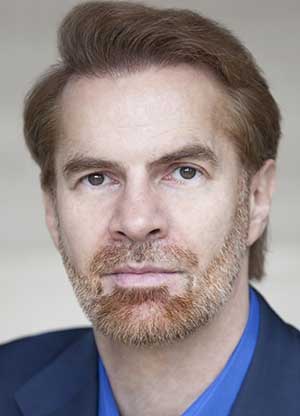
Erik Brynjolfsson says the “Second Machine Age” is already in its second wave.
Visual: MIT Initiative on the Digital Economy
Just how fast this would happen surprised even the authors. Not so long ago, it was common to hear AI disparaged for overpromising. But lately, the headlines have been full of advances like Google’s AlphaGo, a machine-learning platform that beat the world champion of the ancient Chinese game Go a decade before experts predicted it. The accuracy of speech recognition technology has been advancing at a breakneck pace.
These kinds of changes led McAfee and Brynjolfsson, co-founders of IDE, the MIT Initiative on the Digital Economy, to write a follow-up, “Machine, Platform, Crowd: Harnessing Our Digital Future.” The new book asserts that we have entered the “second wave” of the second machine age — a period in which AI, software platforms such as Lyft and Airbnb, and crowd intelligence have become integral to our economy.
For this installment of the Undark Five, I spoke with Brynjolfsson about how software platforms are shifting ideas about traditional business models, how far AI and machine learning have come, and what obstacles remain. Here is our conversation, edited for length and clarity.
UNDARK — The huge valuation of companies like Uber and Airbnb shows that the “platform” concept — creating an app, as opposed to a physical product — has taken hold as a business model. What are the main characteristics of the shift from product to platform?
ERIK BRYNJOLFSSON — This is a revolution we’re seeing in lots of industries. Five of the biggest companies [Alphabet, Apple, Microsoft, Amazon, and Facebook] are now platform companies, but it’s happening all over the economy. Because of digital technology, it’s become easier to connect buyers and sellers and other groups that want to coordinate, and that’s led to more efficient use of a lot of resources, like cars and rooms where people can stay. A lot of those assets have been underutilized, and so now wasted capacity can be matched to consumers who are interested. For instance: A typical automobile is parked or otherwise unused about 95 percent of the time. If you can have that car used, say 50 percent of the time, you’ve got a 10-fold improvement in capital efficiency.
It’s a big opportunity. Every company should be thinking about whether they can either create a platform or be part of platforms, or both. Research by Jean Tirole, a Nobel Prize winner, and Marshall Van Alstyne at the IDE, on the economics of two-sided networks, shows that you get this positive feedback, or “flywheel effect.” As more people join the platform, it becomes more beneficial, and that attracts people on the other side of the network as well.
UD — You and McAfee write that AI is suddenly “over-delivering.” How is AI exceeding our expectations? And why haven’t we been prepared?
EB — There’s a specific change in the paradigm for how a lot of digital technologies and software have been developed. There’s a been a multitrillion-dollar industry based on us coding or codifying our knowledge — in coding what we know, and putting it into computer programs. If you write a tax preparation program or most other programs, you have to know exactly what you want it to do, step by step, and programmers write that software to get the machines to do what they want. But a lot of problems can’t be specified by programmers that precisely because we don’t know how to write all the steps. In the second wave of the second machine age, machines are learning how to figure out the right steps on their own.
Machines do that by having lots and lots of examples of what works and what doesn’t work. “This is a cat.” “This is not a cat.” “This is fraud.” “This is not fraud.” Depending on the examples, they can learn how to solve those problems going forward.
People have been playing around with this for decades, but it’s just recently that there’s been a critical mass of improvements in computer power, data, and improvements to algorithms. Those three things have led to an inflection point where it’s really exceeded the expectations of a lot of the people who had been working on it.
UD — There’s been tremendous anxiety over which human jobs or tasks will be replaced by AI. You write that organizations sometimes over-rely on human judgments and could be better served by tapping into machine intelligence. Can you explain this?
EB — Humans have some cognitive flaws that are well understood. Researchers like Danny Kahneman and Amos Tversky have shown that we humans are susceptible. Just as we have a blind spot in our vision system that you don’t realize is there until someone shows you the blind spot, we have the equivalent of blind spots and biases in some of our decision-making. But now that we have a lot more data available, data-driven decision-making is becoming much more feasible. The evidence shows that companies that adopt this data-driven approach are about 5 percent more productive than those that don’t.
UD — You write that machine learning — a subset of AI in which machines learn to make predictions based on a huge amount of data — can spread quickly, but unevenly. Why is that?
EB — We’re still in early days of this machine learning revolution. In many cases there’s been almost a winner-take-all phenomenon, where the best companies have had huge payoffs. Not everyone understands the opportunity or has access to the right kinds of machine learning or data science experts and algorithms, but I see it diffusing relatively quickly.
So far, most of the successes have been in supervised learning, in which we show the machine learning algorithm lots of examples of what you want and what you don’t want. We haven’t had as much success with unsupervised learning — where the machines go out and look at examples and then they start making their own categories. If they see enough examples of animals, they may notice that some of them kind of have a similar shape and can call those “cats.” And others look a little bit different, and could label those “dogs” — but the humans wouldn’t tell the machine in advance. That’s a lot harder.
UD — What do you see as the overall impact AI will have on the workforce, and how can we prepare for these changes?
EB — As artificial intelligence becomes more powerful, it will inevitably lead to profound changes in our economy and society. Some of these are already beginning, as AI affects an increasing number of tasks and jobs. Over the next 10 years, more kinds of work will be transformed. This will reduce demand for many different skills, like the people working in call centers and driving trucks, and increase demand for other kinds of skills like creative work and interpersonal and caring professions. I see this as the greatest challenge for our society over the next decade.
In the longer term, we will face other types of challenges, but for now I think we should focus on finding ways that we can use technology to create widely shared prosperity that benefits the many and not just a few. This involves rethinking our educational system; boosting entrepreneurship, so we can invent more new products, services, and jobs that will employ people; making the tax system less skewed towards the wealthy; and making new investments in research and development and infrastructure. Ultimately, I think these technologies can be the best things that happened to our society, if we make the right complementary investments and rethink our organizations and institutions.
Hope Reese is a writer and editor in Louisville, Kentucky. Her writing has appeared in Undark, The Atlantic, The Boston Globe, The Chicago Tribune, Playboy, Vox, and other publications.
UPDATE: An earlier version of this piece cut a key part of Brynjolfsson’s response to the question on “over-delivering.” The missing context on machine learning has been added back in.
Comments are automatically closed one year after article publication. Archived comments are below.
Ultimately we going towards a new system of world society where human being will be engaged in creative work and repetitive work will done by machine. Commodity and profit motivated economy is barrier to achieve this. So we should unite to build a borderless cooperative world based on common global ownership of all means of automated production.