Facing Facts: Artificial Intelligence and the Resurgence of Physiognomy
On the first day of school, a child looks into a digital camera linked to the school’s computer. Upon a quick scan, the machine reports that the child’s facial contours indicate a likelihood toward aggression, and she is tagged for extra supervision. Not far away, another artificial intelligence screening system scans a man’s face. It deduces from his brow shape that he is likely to be introverted, and he is rejected for a sales job. Plastic surgeons, meanwhile, find themselves overwhelmed with requests for a “perfect” face that doesn’t show any “bad” traits.
This dystopian nightmare might not be that farfetched, some academics warn, given the rise of big data, advances in machine learning, and — most worryingly — the current rise in studies that bear a troubling resemblance to the long-abandoned pseudoscience of physiognomy, which held that the shape of the human head and face revealed character traits. Modern computers are much better at scanning minute details in human physiology, modern advocates of such research say, and thus the inferences they draw are more reliable. Critics, on the other hand, dismiss this as bunkum. There is little evidence linking outward physical characteristics and anything like predictable behavior, they note. And in any case, machines only learn what we teach them, and humans — rife with biases and prejudicial thinking, from the overt to the subtle and unacknowledged — are terrible teachers.
Still, the research continues.
Just one year ago, engineering professor Xiaolin Wu of McMaster University and Xi Zhang of Shanghai Jiao Tong University jointly published a paper at ArXiv.org, a popular online repository of unreviewed science papers in various stages of development, suggesting that it was possible for a computer to predict who might be a convicted criminal based solely on scanned photographs. (The study has since been taken down, but the authors posted a response to critics here). Wu followed with another study that said machine learning could be used to infer the personality traits of women whose pictures were associated with different words — coquettish and sweet, for example, or endearing and pompous — by a group of young Chinese men.
While the abstract noted that the algorithm is only learning, aggregating, and then regurgitating human perceptions, which are prone to errors, the authors also suggest that “our empirical evidences point to the possibility of training machine learning algorithms, using example face images, to predict personality traits and behavioral propensity.”
In early September, Michal Kosinski and Yilun Wang, both of Stanford University, released a pre-print of a study suggesting that it was possible to detect whether a person was gay or not from photos used on a dating site. The paper was submitted to — and accepted by — the well-respected Journal of Personality and Social Psychology, although this fall, in response to public outcry, the journal’s editors decided to give the paper another look, including what was described as an ethical review.
For all the current controversy, the underlying assumptions of this kind of research are nothing new.
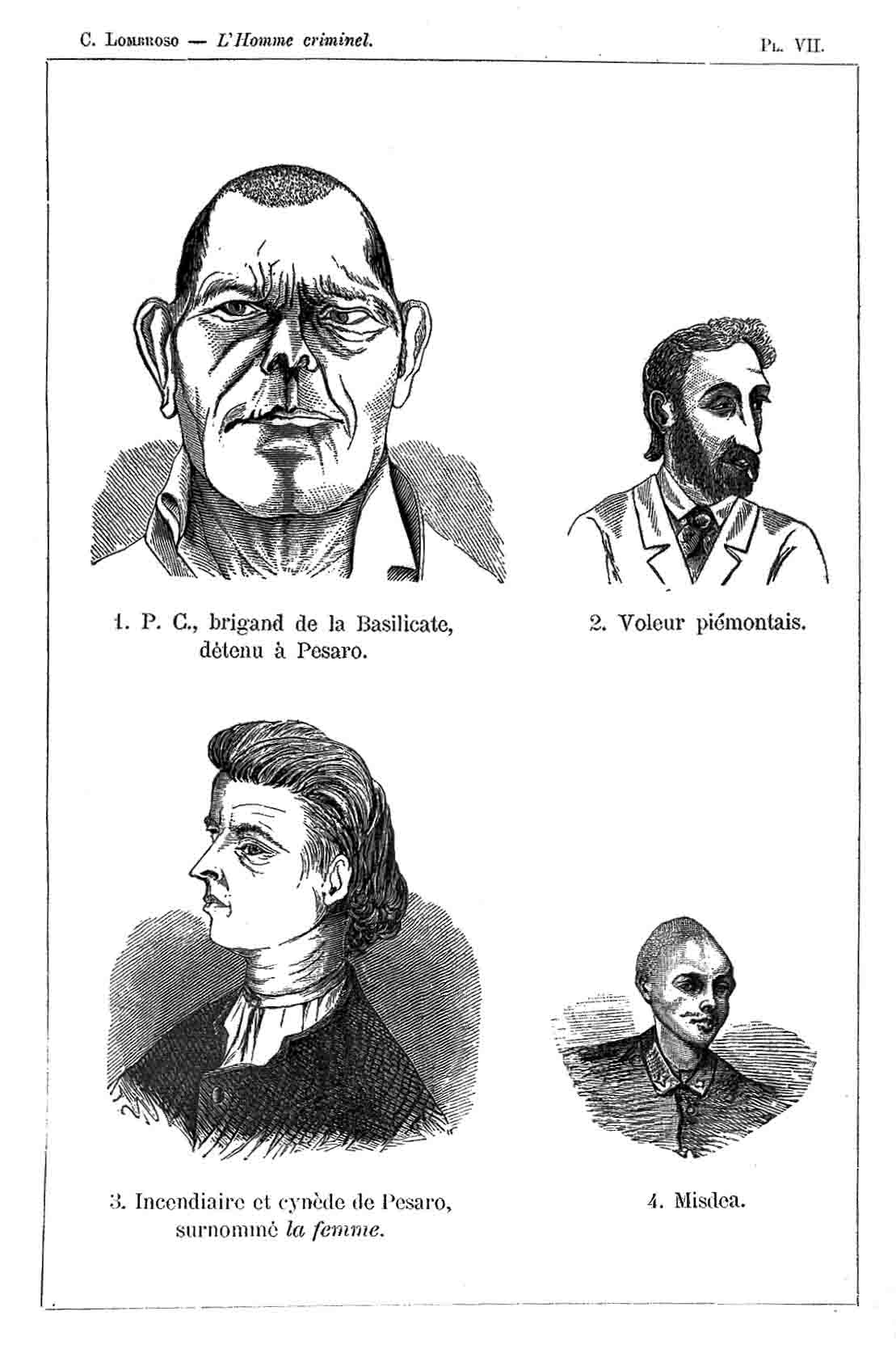
Criminals from Cesare Lombroso’s “The Criminal Man”
Visual: Wellcome Library, London/Creative Commons
The origins of physiognomy trace back to ancient Mesopotamia, but it was in the mid-19th century that Italian criminologist Cesare Lombroso aimed to use it to detect criminality. Lombroso believed that criminals could be spotted by anomalies in facial structure — basically, that they were “throwbacks” and would have “atavistic” features such as a sloping forehead or facial asymmetry. His ideas were abandoned after World War II, but some scientists have continued to seek out correlations between the body and the mind.
Many of those studies deal with human perception — whether we react differently because of what we see, even if that perception is inaccurate. Others look for morphological differences between humans, seeking indications of traits such as aggression, introversion, or homosexuality. Several studies, for example, have indicated the ratio of face height to width — a metric that can be mitigated by differing levels of testosterone — might provide clues about a person’s tendency toward aggression.
But with the rise of huge datasets and advanced computing power, some scientists and psychologists have come to believe that “neural nets” — the networked systems used in machine learning — can analyze physiological and psychological traits in a less biased way than ever before. And where once scientists could study only a few factors, by showing pictures to undergraduates, for example, and measuring a few dozen people’s faces, the internet has opened up an endless trove of inputs. Facial recognition software can take measurements of thousands, even millions of people using photos taken from the web, allowing them to get more — and hopefully better — data.
That information, proponents say, can be fed into machines capable of detecting the most minute patterns, and pick up on physical differences that a human observer could never see.
It sounds fascinating, but to many critics, this is just plain old bad science hiding beneath the veneer of mathematics — algorithms instead of men with calipers measuring noses and brows. And they cite an endless parade of concerns about ethics, privacy, and the introduction of numerous potential biases, intentional or not, into the algorithms that might one day, with a single scan, determine our fates.
While few scientists dispute that physical characteristics can sometimes reveal something about the internal hard-wiring of a person — the testosterone-face connection, for example — the inferences that can be drawn from such observations are vanishingly small. Lisa DeBruine, who co-heads the Face Research Lab at the University of Glasgow’s Institute of Neuroscience and Psychology, describes herself as an evolutionary psychologist, but she is not shy about the shortcomings in the field. As for width-to-height ratios, “That area of research is a real mess,” she said, largely because of inconsistencies in how both humans and computers measure things like the distance from a chin to a brow or cheekbone to cheekbone.
DeBruine was one of many critics of Kosinski’s study, which sought to train a computer to identify who was gay and who was straight, based solely on scans of faces from a dating site, where users clearly identified their sexual orientation or preference. Kosinski claimed that, given two new photos, one of a straight person and one of a gay person, the computer was able to guess with a high degree of accuracy which one was gay. He also tested the algorithm on Facebook photos the computer had never seen, and it seemed to get it right — at least for gay people. The algorithm seemed to do less well identifying straight people.
(This may sound counterintuitive, but the algorithm wasn’t using the process of elimination. Rather, when given the binary choice of identifying a single person in a photograph as being straight or gay, it seemed to get it right less often when presented with a straight person, assigning them as gay when they were not.)
In his paper, Kosinski said the computer was zeroing in on certain physical features of the face consistent with prenatal hormone theory (PHT), which suggests that gay people are so because they were exposed to differing levels of androgens in the womb. “The faces of gay men and lesbians had gender-atypical features,” the scientist wrote, “as predicted by the PHT.” Kosinski argues that prenatal hormone theory is “widely accepted” as a model for the origin of homosexuality.
Critics, though, said there are several problems with Kosinski’s formulation, including a variety of stereotypical assumptions, and a failure to appreciate that PHT isn’t necessarily as solid as it sounds. The computer might also be picking up on something besides a feature set that defines gayness.
Greggor Mattson, an associate professor of sociology at Oberlin College, said the idea that testosterone exposure makes people gay in the womb depends in part on a stereotype of gay men as feminized and lesbians as masculinized women. “His work reinforces tired stereotypes and bad science about sexuality,” he said in an email message. The data science in itself is not the problem, he suggested, but how one interprets that data.
Philip N. Cohen, a sociologist at the University of Maryland, noted in a blog post that Kosinski’s work isn’t inconsistent with PHT, but it doesn’t provide much support for it either. And Carwil Bjork-James, an anthropologist at Vanderbilt University, noted that PHT theory is far more debatable than Kosinski suggests.
According to Bjork-James, Kosinski’s chief problem is failing to appreciate a variety of confounding factors. For example, the photos on a dating site might not be representative. “People choose photos,” he said. “If I am going to put something on a gay dating site, I will use the one that’s ‘most gay.’” Who is gay or not, and how that gets transmitted, is a complex interplay between presentation and perception by other people. But Kosinski and his team “skip to prenatal hormone imbalance theory,” Bjork-James said.
Kosinski maintains that the study wasn’t testing PHT, only whether an algorithm could be used to “out” gay people, and that he wants to warn people about such technologies. “Look — in the context of a person, country or institution that wants to ‘out’ people, it’s just a great pre-screening tool,” he said.
Yet even Kosinski admitted that the computer might be picking up something besides immutable facial features. His algorithm, for example, posits that gay men are more likely to wear glasses. “Many wondered why faces with glasses are considered by algorithm to be more likely to be gay,” Kosinski said. “It might be something else in the face that’s also correlated with having glasses.”
Wu’s detractors also noted similar problems in the data used to train computer algorithms purporting to identify criminals. His work used identification photos for the convicted criminals, and pictures taken from web searches for the non-convicted set. But Carl Bergstrom, a professor of evolutionary biology at the University of Washington, and Jevin West, an assistant professor in the Information School at the university, said in their evaluation of Wu’s work that it’s possible that the artificial intelligence algorithm was finding small differences in expression. (Wu acknowledged this in his open letter to his critics).
Still, even if there really are physiological differences between the faces of criminals pictured in photographs and the non-criminals fed into the computer, that may simply reveal more about stereotypes within the Chinese criminal justice system than the people in the photos. Critics have also noted a lack of transparency in the origin of the photo set for the convicted criminals. (Wu says in the study that some are from a police department in China with which he signed a nondisclosure agreement.)
Wu’s follow-up study on the reactions to attractive female faces has a similar problem, Bjork-James suggested. It might be a good study of the stereotypes of Chinese men, he said, or even the effect that women are trying to achieve for Chinese men, but it says nothing about the behavior or personalities of the women.
In an email message, Wu seemed to agree. “Our study was on the perception of socio-psychological perceptions of faces only,” he said. “It concludes NOTHING about the validity of these perceptions predicted by our AI algorithms.”
Scientists aren’t the only ones getting into physiognomy. An Israeli startup, Faception, advertises the ability to spot pedophiles and terrorists using face recognition technology by looking for certain traits. Affectiva, a Boston-based firm, offers a face recognition system that it says can detect emotions to aid advertisers.
Jonathan Frankle, a Ph.D. student at MIT who served as the staff technologist at Georgetown University’s Center on Privacy and Technology, said we often don’t know how these sorts of algorithms work. Unlike ordinary code, a neural net involves nodes that are interconnected, with processes happening in parallel. A neural net isn’t just executing a set of instructions in sequence; instead the nodes are talking to each other, giving feedback to each other. There really isn’t any way to trace how it makes its decisions — one can’t look at a single line of code or subroutine. It’s effectively a black box.
That means it is far from clear that the algorithms and artificial-intelligence engines we design are picking up on the features we think they are, and Kosinski’s study reflected this: When he tried to create a composite “gay” face for men, it had eyeglasses, but there is no evidence gay people have more vision problems than the population at large. Kosinski said it could be a fashion choice among gay men, but this undercuts the idea that there is something different in the morphology of gay faces.
Not knowing what the AI is doing is particularly worrisome when a startup is advertising that it can detect threats, as Faception does. Citing the need to keep trade secrets, the company’s CEO, Shai Gilboa, would not say exactly how its algorithm is designed, or what kind of data they used to train it. But he did emphasize that Faception’s systems were based on solid research, and that they are unbiased. “It’s very important from an ethical point of view,” he said. “We prove repeatedly we have no measures of color or race.”
Affectiva, meanwhile, doesn’t say it can detect anything about innate personality traits from facial structure, just the emotional state of a person. Further, CEO Rana el Kaliouby adds that her company made a point of training its AIs on people from many countries and using those populations as points of comparison (so, for example, a version of the software running in China or Brazil would be trained using images of people from that country).
It’s a way to avoid missing cultural differences in the circumstances in which people display emotions, el Kaliouby said. And crucially, she says that Affectiva’s team interrogated the AI to be sure they knew what it was picking up on when reading facial expressions.
Affectiva claims its technology can discern a person’s ‘moment-by-moment emotional state’ by monitoring their facial expressions.
For all the technical concerns, critics say there are the ethical questions, too. Kosinski’s study used photos without the consent of the people in them, and he did not work closely with any organizations that represented gay people. (GLAAD, one of the more prominent LGBTQ rights organizations in the U.S., released a joint statement with the Human Rights Campaign blasting the study’s methods.) In Wu’s case, the photos of convicted criminals were provided by a Chinese police department, though he said he could not name it. Both studies raise concerns about what happens if some government or private company decided to use these techniques with discriminatory intent from the start.
But it’s really the raft of tenuous, culturally constructed assumptions that go into building algorithmic facial analysis that are most worrying, critics say. “Social psych has a serious native-English speaking, cis, straight, white guy problem (like many fields), which definitely leads to these sorts of studies,” DeBruine said in an email. “I can’t imagine [Kosinski’s] study would have gotten as far as it did if there were more diverse people in positions of influence at some point in the pipeline (colleagues, ethics committees, the editor, reviewers, etc.)”
To that extent, Frankle is happy that such studies occur in the open literature. “I would rather it be done in the open by academic researchers than by a private company and sold to some police agency,” he said.
Clare Garvie, an associate at the Center on Privacy and Technology at Georgetown Law, said another problem with these studies isn’t always the science, questionable as it is, but that humans tend to believe computers — or anything that sounds like it is backed up by math. “These kinds of tools can determine who comes into a country or gets a job,” she said. Faception’s claims might be bolstered if Kosinski’s work — or anything like it — gets published in respectable journals. The fact that Faception’s marketing materials include identifying a terrorist by a knit cap and a goatee doesn’t raise Garvie’s confidence.
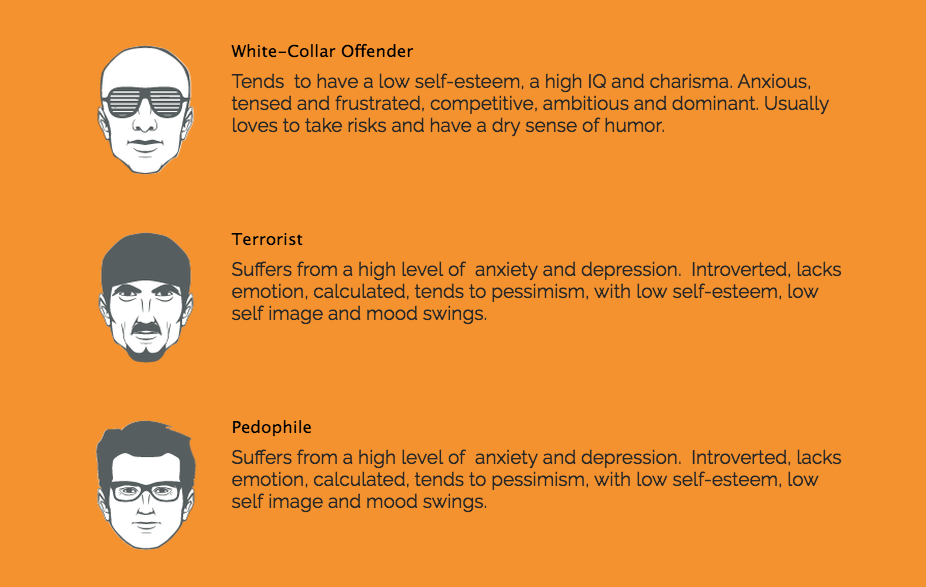
Three of eight “classifiers” illustrated on Faception’s website.
Visual: Faception
For his part, Gilboa said the website images were simply a design decision, and he provided several studies of the relations between facial symmetry and personality. Some of those focused on marketing and advertising. Another covered the geographic distribution of personality types. None of the studies addressed Faception’s claims directly.
Fixing any of these problems isn’t impossible, and most of the experts interviewed agreed this kind of research can be done better. Walter Scheirer, an assistant professor of computer science at Notre Dame, for example, said diversifying the people in the computer science field can help, as would a broader dialogue between computer science and other disciplines.
Mark Frank, a professor of communications at the University at Buffalo in New York, added that a bit of humility and skepticism would help, too. “The computer scientists’ understanding of behavioral stuff is charming, is the word I will use,” he said. There’s just so much more complexity to the phenomena they are measuring, Frank said, and any connections between physicality and behavior are likely to be far more subtle — and far more subject to myriad confounding factors — than these initial studies suggest.
Bergstrom, the evolutionary biologist, suggested that all of this springs from a longstanding, if errant, desire to find psychology encoded in the body. “I do think there’s this very long-running notion in human thought that our external appearance reflects our internal mind and morals,” he said. That idea gets regenerated over time, he said, “and we can trace that back for millennia.”
Jesse Emspak is a freelance science writer whose work has appeared in various outlets, including Scientific American, The Economist, and New Scientist. His focus has been the physical sciences and the knock-on effects of technology. In his previous reporting life, he covered finance in New York and London when the dot-com bubble burst. He lives in New York City.
Comments are automatically closed one year after article publication. Archived comments are below.
It may well be the case that we cannot prevent this kind of research (although there is research and funded research in which ethics and intent should surely be considerations…). What concerns me is the persistence of stupid and prejudicial ideas whose sole utility is their applications for purposes of social judgement and control. People keep writing that many of these ideas ‘went away’ after WW2 as though all that murder was a lesson for our societies. But mostly, many eugenicists rebranded themselves as geneticists and carried on with their academic careers. So accountability has not been well served and the various professions that peddle social determinism seem to keep doing so to and for their own benefit. What we need is a more engaged critique of this sort of thing and its almost inevitable consequences.
Jonathan Frankle has the right idea: whether right or wrong, there’s no way to prevent this kind of research and it’s better that it be conducted and publicized in daylight than in the dark. The current and future availability of data on a scale previously impossible makes the potential payoff for success so large that research will continue, like it or not. If it’s socially banned, then we just won’t hear about it until Cambridge Analytica is successfully selling its data for use with saleable versions of these kinds of programs. I’m sure it sounds offensive to many people, as it does to me, but it does no good to rant and rave. As the past half century has shown only too well, If such things can be monetized they will be, one way or another.